WiMi Hologram Cloud Builds Advanced Data Structure Architecture Using Homomorphic Encryption and Federated Learning
Ensuring Data Privacy while Enabling Efficient Data Analysis and Sharing
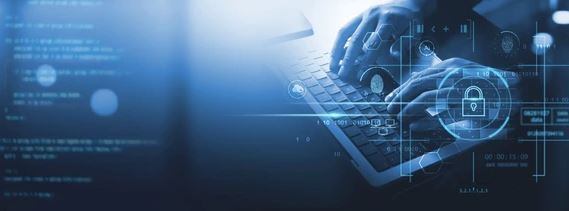
WiMi Hologram Cloud Inc., a leading global Hologram Augmented Reality (AR) technology provider, has announced that it utilised homomorphic encryption and federated learning to build an advanced data structure architecture. The architecture integrates federated learning and partial homomorphic encryption, and this integration protects data privacy while enabling efficient data analysis and sharing.
The China-based company is a holographic cloud comprehensive technical solution provider that focuses on professional areas, including holographic AR automotive HUD software, 3D holographic pulse LiDAR, head-mounted light field holographic equipment, holographic semiconductor, holographic cloud software, holographic car navigation and others.
WiMi Hologram Cloud’s services and holographic AR technologies include holographic AR automotive application, 3D holographic pulse LiDAR technology, holographic vision semiconductor technology, holographic software development, holographic AR advertising technology, holographic AR entertainment technology, holographic ARSDK payment, interactive holographic communication and other holographic AR technologies.
Homomorphic encryption is a special encryption technique that enables computational operations to be performed in an encrypted state without decrypting the data. By utilising homomorphic encryption, it is possible to compute and share data in an encrypted state while protecting data privacy and integrity, which is useful for some scenarios involving sensitive data.
Federated learning, on the other hand, is a distributed machine learning technique that enables model improvement by allowing multiple participants to train models on their respective local datasets without sharing the original data, and aggregating the learned parameters of these models into a global model. In data structuring, federated learning can address the issues of data privacy and data security.
Latest Breakthrough from WiMi Hologram Cloud Enables Secure Data Sharing and More
WiMi Hologram Cloud’s data structure architecture based on homomorphic encryption and federated learning enables data collaboration, sharing, and integration without revealing the original data content. Participants can train models and update parameters without direct access to the original data of other participants, providing an effective and reliable data fusion solution for secure sharing and analysis of big data.
The architecture not only protects the privacy of data, but also improves the efficiency and accuracy of data integration. In practical application, firstly, the requirements of the data architecture need to be analysed in detail, including data type, data size, and computational tasks.
Based on the results of the demand analysis, the design objectives and functions of the data structure are determined. Then, homomorphic encryption technology is utilised to encrypt the user’s sensitive data to ensure that the data remains encrypted during the computation process. The encrypted data from the participating parties are then aggregated and computed using federated learning techniques. The federated learning process can be implemented using secure multi-party computation protocols or differential privacy techniques to ensure data privacy and accuracy of computation results.
The fusion application of homomorphic encryption and federated learning is of great significance in the data structure, which can provide efficient computation and analysis capabilities while protecting user privacy, bringing more possibilities for technology utilisation in the technology industry. This application is expected to play an important role in medical and financial fields, promoting secure data sharing and innovative research, and promoting the continuous development of the big data field.
Compelling Use Cases of WiMi Hologram Cloud’s Innovative Approach to Data
For example, in the medical field, patients’ medical data often involves personal privacy, and how to share and analyse medical data while ensuring data privacy has been a challenge for medical informatisation. WiMi Hologram Cloud’s architecture provides a feasible solution for secure sharing of medical data by combining federated learning and homomorphic encryption. Hospitals and research institutes can work together to train and optimise medical models without disclosing patients’ personal information, improving the quality and efficiency of medical services.
In the financial sector, financial institutions are faced with a large amount of sensitive data, such as customer identity information and transaction records. The leakage of these data may have a serious impact on the reputation of financial institutions. The data structure architecture based on homomorphic encryption and federated learning researched by WiMi Hologram Cloud can help financial institutions improve the accuracy and efficiency of their risk control models and effectively prevent financial risks by encrypting data sharing and analysing them under the premise of ensuring data security.
In addition, with the popularisation of IoT devices and the development of social networks, data generation and sharing have become more and more frequent. How to realise the effective integration and utilisation of data while protecting personal privacy has become an urgent problem in these fields. WiMi Hologram Cloud’s data structure architecture based on homomorphic encryption and federated learning provides an effective solution to these problems.
In the future, WiMi Hologram Cloud will continue to conduct in-depth research and development of data structure architecture based on homomorphic encryption and federated learning and promote the application and popularisation of such architecture in various fields. In the future, this architecture combining federated learning and homomorphic encryption will become an important development direction in the field of big data.