Snowflake Research Reveals that 92% of Early Adopters See ROI From AI Investments
Early adopters are already seeing positive ROI from AI investments, despite ongoing challenges in data readiness and use case prioritisation.
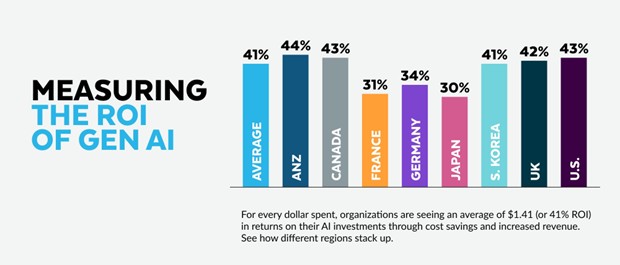
Snowflake, the AI Data Cloud company, in collaboration with Enterprise Strategy Group, today released the “Radical ROI of Generative AI”, a global research report surveying 1,900 business and IT leaders across nine different countries — all of whom are actively using AI for one or more use cases. Of all respondents, 92% reported that their AI investments are already paying for themselves, and 98% plan to invest more on AI in 2025. As AI adoption accelerates across global enterprises, a robust data foundation has emerged as the cornerstone of successful implementation, yet respondents are still grappling with how to make their data AI-ready.
“I’ve spent almost two decades of my career developing AI, and we’ve finally reached the tipping point where AI is creating real, tangible value for enterprises across the globe,” said Baris Gultekin, Head of AI, Snowflake. “With over 4,000 customers using Snowflake for AI and ML on a weekly basis, I routinely see the outsized impact these tools have in driving greater efficiency and productivity for teams, and democratising data insights across entire organisations.”
Businesses Report Varying Levels of AI Success Across the Globe
Early AI investments are proving to be successful for the majority of enterprises, with 93% indicating that their AI initiatives have been very or mostly successful. In fact, two-thirds of respondents are already starting to quantify their generative AI ROI today, finding that for every dollar spent, they are seeing $1.41 in returns (or 41% ROI) through cost savings and increased revenue.
However, there are global nuances around where organisations are focusing their AI efforts that directly correlate to each country’s AI maturity, and their results in terms of driving ROI across regions:
- Australia and New Zealand (ANZ) respondents have seen a 44% return on their AI Compared to the global average, organisations in ANZ were more likely to cite enhancing customer satisfaction as a key goal for their AI initiatives (53% versus 43%), and less likely to prioritise internal-facing projects (47% versus 55%).
- Canada respondents have seen a 43% return on their AI Canadian organisations were more likely to say that they’re only pursuing initial AI use cases (45% versus 36%), suggesting that many are earlier in their AI adoption journeys than global counterparts.
- France respondents have seen a 31% return on their AI investments. Compared to the global average, French companies are less likely to train or augment large language models (LLMs) with proprietary data using retrieval-augmented generation (RAG) (59% versus 71%), suggesting a lag in maturity for their AI strategies.
- Germany respondents have seen a 34% return on their AI German organisations were more likely to report challenges with infrastructure, particularly in meeting storage and compute requirements for AI (69% versus 54%).
- Japan respondents have seen a 30% return on their AI Japanese organisations differed in their strategic goals for AI, being least likely to focus their AI efforts on customer service and support (30% versus 43%) and financial performance (18% versus 30%), but the most likely to harness AI to help cut costs (43% versus 32%).
- South Korea respondents have seen a 41% return on their AI investments. South Korean businesses are employing mature AI use cases, reporting the highest use of open source models (79% versus 65%), and are more likely to train or augment models with RAG (82% versus 71%).
- United Kingdom respondents have seen a 42% return on their AI investments. In terms of strategic goals, UK-based organisations were more likely to prioritise the value AI brings to end users, with respondents beating the global average in citing both operational efficiency (57% versus 51%) and innovation (46% versus 40%) as primary business drivers.
- United States respondents have seen a 43% return on their AI investments. American companies led in successful AI operationalisation, with respondents more often than any other country to say that they’ve been “very successful” at operationalising AI to achieve their business goals (52% versus 40%).
Organisations Face Increased Pressure to Select the Right Use Cases
Despite almost all respondents reporting success with their AI initiatives to-date, many organisations are grappling with difficult decisions to build on the momentum. Amid a sea of opportunities to implement AI within their businesses, respondents reported challenges with identifying the most impactful use cases and increased pressure to make the right decisions — all while grappling with limited resources:
- Too many use cases, too few resources: 71% of early adopters agree they have more potential use cases that they want to pursue than they can possibly fund.
- Decision-making blind spots: 54% agree that selecting the right use cases based on objective measures like cost, business impact, and the organisation’s ability to execute is hard.
- Competitive pressure mounts: 71% acknowledge that selecting the wrong use cases will hurt their company’s market position.
- Job security concerns arise: 59% of respondents say that advocating for the wrong use cases could cost them their job.
Overcoming Data Barriers to Maximise AI Effectiveness
Organisations are increasingly incorporating their proprietary data to maximise AI’s effectiveness, with 80% of respondents choosing to fine-tune models with their own data. Despite this widespread recognition of data’s importance — with 71% of respondents acknowledging that effective model training and fine-tuning requires multi-terabytes of data — significant challenges persist in making this data
AI-ready. With the majority struggling to make use of their most valuable asset, organisations claim that the following are the biggest data hurdles for driving AI success:
- Breaking down data silos: 64% of early adopters say integrating data across sources is challenging today.
- Integrating governance guardrails: 59% say enforcing data governance is
- Measuring and monitoring data quality: 59% say measuring and monitoring data quality is
- Integrating data prep: 58% say making data AI-ready is a
- Efficiently scaling storage and compute: 54% say it’s difficult to meet storage capacity and computing power requirements.
There is a significant opportunity for businesses to overcome these challenges and unlock the full potential of their data for more accurate, relevant, and impactful AI outcomes with a unified data platform.
“The rapid pace of AI is only accelerating the need for organisations to consolidate all of their data in a well-governed fashion,” said Artin Avanes, Head of Core Data Platform, Snowflake. “Having an easy, connected, and trusted data platform like Snowflake is imperative not just for helping users see faster returns on their data investments, but it lays the foundation for users to easily scale their AI apps in a compliant and secure manner — without requiring specialised or hard to find technical skills. A managed, interoperable data platform provides seamless business continuity as global enterprises tap into their entire data estate to lead in the evolving AI landscape.”
Learn More:
- Read the full research report, “Radical ROI of Generative AI,” and double click into the findings in this blog post.
- See how Snowflake is providing thousands of users with a robust platform that is easy to use, connected, and trusted in order to support both current and future AI needs in this blog post.
- Learn more about how global organisations can get started with AI data agents today and define an ROI framework to measure business impact in this A Practical Guide to AI Agents ebook.
- Stay on top of the latest news and announcements from Snowflake on LinkedIn and Twitter / X
Methodology
Researchers from Enterprise Strategy Group identified, and conducted deeper research between Nov. 21, 2024, to Jan. 10, 2025, with early adopter organisations — those already augmenting and executing
business processes in production, using commercial and open-source models rather than
consumer-grade, subscription software such as ChatGPT. Of 3,324 respondents, 1,900 (57%) said they are using commercial or open source generative AI solutions. Additional details around methodology can be found within the report.