Ethical AI Considerations: Scalable Data Solutions Are Critical to Navigating Asia’s Fluid AI Terrain
AI Is a Double-Edged Sword, and Managing Data Sets Is Necessary to Use It for Good
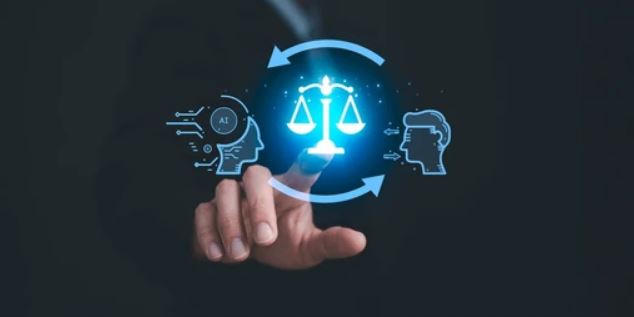
By Jihad Dannawi, General Manager, APAC, DataStax
Understandably, many are preoccupied with levelling up the customer experience, elevating efficiency, and empowering employees to be their best, most productive versions of themselves. No wonder, then, that many companies are looking to leverage Artificial Intelligence (AI)—yet are maybe forgetting about ethical AI.
In Southeast Asia, for example, Bain and Co. found that AI played a significant role in raising the region’s revenues from the digital economy to USD $100 million last year. These numbers strongly suggest rising adoption, which is also borne out elsewhere in Asia.
For instance, IDC notes high hopes for GenAI outcomes in product development/design, research and development, customer engagement, sales, and marketing in most of the Asia Pacific.
However, how many business decision-makers actually consider the ethical implications from all angles? After all, AI also comes with its own share of risks—ranging the gamut from deepfakes to copyright infringement—if ethical AI is not observed.
Generative AI, specifically, has also shown itself to be prone to making up answers—or “hallucinating”—to respond to prompts it does not know how to answer. There’s also growing concern about biased data and its implications. While that problem is a question of data quality, ensuring data security is also a key imperative for implementing AI. Ensuring data privacy and security and ethical AI is crucial, especially for sectors like finance or healthcare, where organisations hold onto vast troves of sensitive information.
Let us also not forget that people are central to AI success. That means equipping employees with the skills to leverage AI tools. In sum, businesses are facing a proverbial minefield. Truly leveraging AI, therefore, hinges on a holistic strategy that prioritises ethics on par with the bottom line and efficiency.
Tackling Bias and Ensuring Ethical AI via Robust Governance
One thing that is fundamental to approaching AI is to recognise that these machines only know what their algorithms and data sets allow them to. If those same algorithms and data sets have not been vetted for biases to begin with, AI does not have the wherewithal to recognise and counter them. And this unconscious perpetuation of inaccuracies is a critical problem today. A NIST report, for example, highlighted that despite systemic institutional and social factors fuelling AI bias, the issue itself was being overlooked.
Overcoming that, then, rests on expanding perspectives to extend past machine learning pipelines. That begins with AI governance policies, which IBM notes, encompasses the following:
- Ensuring compliance so that AI solutions align with industry regulations and legal requirements.
- Prioritising data protection via trustworthy AI to safeguard customers’ interests.
- Acting transparently by shedding light on data used and what processes underpin AI-powered services.
- Adopting methods like counterfactual fairness to ensure equitable outcomes regardless of sensitive attributes.
- Instrumentalising “human-in-the-loop” systems to ensure people still oversee AI and maintain quality assurance standards.
- Reinforcing learning to overcome human biases and discover innovative solutions.
Accounting for the Emergence of Dark AI
You may be familiar with the Dark Web, and in these rapidly evolving times, we are now approaching an era of Dark AI. As the name suggests, these large language models (LLMs) are essentially being used by malicious actors who want to harness the power of AI for crime, misinformation, and so on. This only highlights the importance of ethical AI even more.
Make no mistake, its emergence is poised to bring about disruption in societal and/or business realms. They currently can take the shape of automated agents, fuelled by open-source and unmonitored LLMs. These Dark AI tools hold the potential for extensive misuse and could be utilised for a spectrum of nefarious activities ranging from financial fraud and organised crime to bioterrorism and terrorism.
“Understandably, many are preoccupied with levelling up the customer experience, elevating efficiency, and empowering employees to be their best, most productive versions of themselves. No wonder, then, that many companies are looking to leverage AI—yet are maybe forgetting about ethical AI.”
Furthermore, LLMs introduce a new avenue for exploitation by malicious entities, including the creation of highly convincing and personalised phishing emails, production of deepfake videos, automation of illicit financial transactions, or dissemination of detailed plans for unlawful or terrorist acts.
Yet, not all is lost. LLMs can also be leveraged for good and ethical AI, and there are avenues for LLMs to be used to bolster cybersecurity. By analysing vast amounts of data, LLMs can identify patterns and detect anomalies that signal cyber threats, thus enhancing security measures.
However, to effectively harness the power of LLMs for cybersecurity, organisations across Southeast Asia and beyond must equip themselves with scalable data solutions. Scalable data solutions enable the processing and management of large datasets efficiently, providing the necessary infrastructure to support the advanced capabilities of LLMs.
This is ultimately the only way to have a comprehensive solution for ethical AI use cases—as it delivers extensive data resources and provides a fully furnished infrastructure characterised by high relevancy and minimal latency. These are core ingredients to leveraging the powers of AI, such as retrieval augmented generation (RAG). That, then, positions organisations to build ethical AI with a strong governance structure, ensuring organisation-wide understanding of AI limitations that will help prevent misuse down the line.