Data Annotation as the Lifeblood of AI Models In the Eyes of TDCX and SUPA
AI specialists share their thoughts on why data annotation is the key to building accurate, ethical, and secure AI systems.
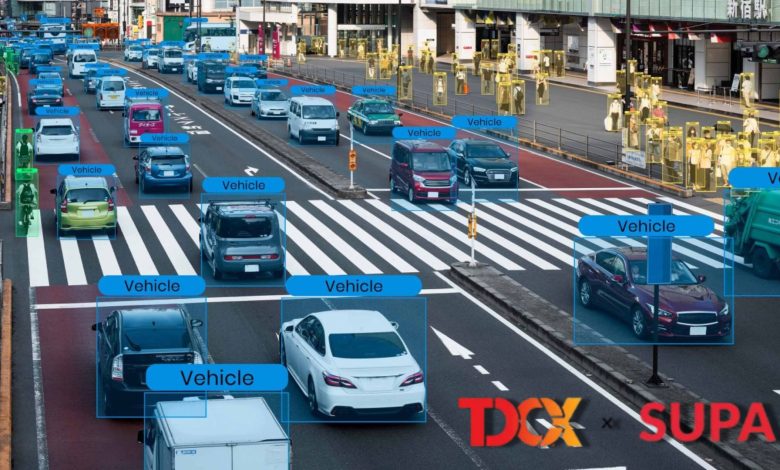
Clean and well-curated data is the backbone of reliable AI models, yet data annotation remains one of the most time-consuming and costly aspects of AI development.
According to CloudFactory, businesses investing in AI often find that up to 80% of project time is consumed by data preparation, including labelling and curation. With public datasets becoming increasingly scarce, companies must harness their own private data while ensuring security, compliance, and accuracy.
In an interview with Lianne Dehaye, Senior Director at TDCX AI, and Mark Koh, CEO and Co-founder of SUPA, they discuss the importance of clean data, the evolving AI landscape, and how businesses can bridge the gap between automation and human expertise in data annotation.
Trends Shaping AI Development
The adoption of AI models is accelerating across industries, particularly in the APAC region, where at least 16 jurisdictions are implementing or considering AI governance frameworks.
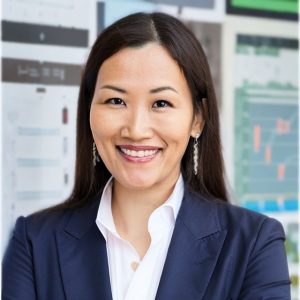
Dehaye notes that ethical AI development is becoming a priority, with businesses focusing on fairness, bias mitigation, and human oversight. âThis shift will heighten the importance of building a team of experienced annotators with subject matter expertise or outsourcing data labelling to ensure high-quality, unbiased data labelling,â she said.
Meanwhile, Koh highlights that as more businesses build their own AI models, they are beginning to realise the critical role of well-trained annotators. âAn untrained or poorly managed labelling team can introduce bias into AI models, leading to flawed outputs that fail to meet the needs of end-users,â he explains.
Koh also mentioned that this âbias creepâ can result in unreliable AI applications, making the need for precise data labelling even more pressing.
Traditional Industriesâ Machine Learning Adoption
Industries that have historically not relied on itâsuch as manufacturing, healthcare, and agricultureâare beginning to integrate AI-driven solutions.
From automating production lines to AI-generated art, the technology is reshaping traditional sectors. However, the rapid adoption of AI also raises concerns about intellectual property, misinformation, and the need for human oversight.
Dehaye notes that AI-generated content is increasingly influencing creative industries, pushing the boundaries of human expression. âAI-generated videos, music and literature are pushing the boundaries of human creativity, inspiring new forms of expression and collaboration,â she said. âHowever, that also means creators will have to grapple with challenges, such as intellectual property and copyright concerns from consumers.â
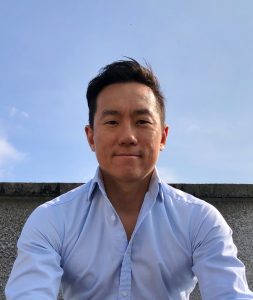
In the aspects of industrial sectors, Koh hails AI adoption as a transformative movement to the industry. He credited their portfolio as an example, saying, âWe worked with a global consumer electronics company to improve defect detection on production lines. By refining the labelling process and deploying experienced annotators, we achieved a 96% accuracy rate over time.â
But just like Dehaye, Koh also emphasises humanâs capabilities in managing the technology, stating that while automation speeds up workflows, human expertise is still essential for ensuring quality control.
This hybrid approach of leveraging AI for efficiency and human oversight for precision demonstrates the role of AI across industries that previously operated without it.
Why Clean Data is Essential for AI Models
Poor data quality can significantly impact AI performance, leading to inaccurate predictions and unreliable decision-making.
Dehaye cites the case of autonomous vehicles (AV), where precise data labelling is crucial for safety. âData labelling requires precise classification of critical objects, such as bicycles versus motorcycles, or construction barriers versus traffic cones,â she said. âMislabelling could result in potential accidents or liability issues, such as a yield sign that caused an AV to slow down instead of coming to a complete stop of intersection.â
She provided these examples to highlight that while AI can perform data labelling work to some degree, it will always come back to human oversight capabilities, to ensure accurate labelling and verification.
Koh echoed the same sentiments, stating that curated data is the true essential of successful AI models as it ensures the accuracy, relevance, and reliability of the insights these models produce.
Koh explained, âAs AI adoption expands into regions like Southeast Asiaâwhere linguistic, cultural, and contextual nuances are often underrepresented in globally trained models such as ChatGPT, Gemini, or Llamaâthe demand for well-curated, localised data becomes even more critical.â He further elaborated that localised data help fine-tune models to better understand and respond to regional languages, dialects, and cultural contexts.
Ultimately, both AI experts emphasised the critical role of clean data. Proper labelling and data organisation help prevent AI models from generating misinformation, which can lead to harmful outcomes for the public and damage business reputations.
Security and Ethical Considerations in Data Labelling
Data security is a growing concern in AI development. Companies must protect raw training data while ensuring that labelled datasets remain secure.
Dehaye stressed that organisations need a combination of technical measuresâsuch as encryption and access controlsâalong with operational safeguards, including regular security audits and background checks on personnel handling sensitive data.
Koh adds that companies should adhere to security standards like General Data Protection Regulation (GDPR), SOC 2 Type 2âan auditing procedure that ensures your service providers securely manage your dataâand ISO/IEC27001. âPlatforms offering robust security features, such as transparent audit trails and secure data handling practices, are critical in protecting AI models from potential vulnerabilities,â he says.
Overall, human competencies and regulatory compliances are the two important matters every organisation must juggle to ensure seamless security over their data resources. With threat actors increasingly evolving their methods, the stakes in securing respective resources have become higher than ever.
The Future of Data Annotation
With AI evolving rapidly, businesses must rethink how they handle data annotation to stay competitive.
Dehaye believes that companies must carefully decide which data labelling tasks can be automated and which require human expertise. âA strong feedback loop between AI and human annotators can drastically improve accuracy while ensuring that AI models remain ethical and bias-free,â she notes.
SUPA and TDCX have collaborated to enhance data annotation efficiency by integrating AI automation with human-in-the-loop processes. âBy combining AI-driven labelling with human validation, we help businesses achieve faster, more accurate data labelling while maintaining high-quality standards,â Koh explains.
With a well-balanced approach, businesses can overcome challenges in adopting cutting-edge technologies like AI, and position themselves for long-term success.