Navigating Social Media Marketing: The Challenges of Sentiment Analysis
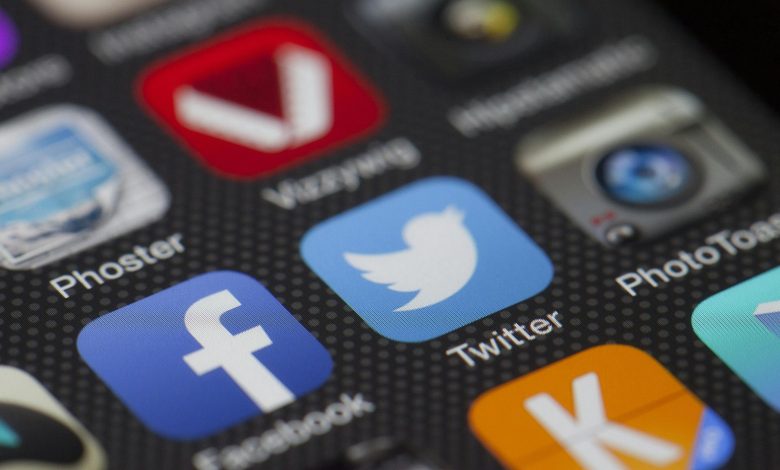
By Associate Professor Dr. Nan Jiang of Taylorâs Business School and Professor Jason James Turner of Southampton Malaysia Business School
Social media has become an integral part of daily life in Malaysia, with widespread usage across the nation. As of June 2024, the number of active social media users in Malaysia was around 31.95 million, representing approximately 83.10% of population in Malaysia. This figure is expected to grow by an additional 7.77% by 2029, reaching 34.68 million users. Currently Facebook has the largest share (68%) of the Malaysian social media market, followed by X (formerly known as Twitter) and Instagram.
The reason(s) behind the popularity of social media is arguably because of its ability to connect people, providing platforms for expression and tailored content to provide an engaging user experience. Supporting the various interfaces is social media analytics which enhance business intelligence and decision making, spanning across disciplines such as digital marketing, economics, fintech and computer science.
The most commonly used techniques for social media analytics are text mining and sentiment analysis. Both techniques deal with textual data, typically involving the extraction of underlying patterns or insightful information from various textual data sources. Sentiment analysis emphasises the extraction of more emotional content from textual data and categorises outputs into predefined mutually exclusive categories by adopting polarity of opinion (positive, negative and neutral emotions) and then presenting numeric codes of statistical analysis. In the context of digital marketing, sentiment analysis plays a crucial role in market intelligence, content strategy, customer engagement, competitive analysis, optimising brandings and trend forecasting.
Despite the potential benefits of sentiment analysis, there are several challenges associated to its usage, especially in relation to analysing user-generated textual content. The first challenge is multifaced object identification, feature extraction and opinion grouping. Sentiment analysis only extracts and classifies accordingly to a studyâs focal objects. While the comments or posts expressed in social media may be derived from various backgrounds, demographics and often contain multiple phrases, symbolic markers (e.g. hashtags â#â, punctuation marks, â???â, ââŠ!!!â, abbreviations, âLOLâ, âBRBâ), emojis (đąđ), wordplay (e.g. â2morrowâ and âgood 9â) and slang (e.g. âgoatâ, âghostâ). Such diversity and richness in the textual data could increase the complexity for social media analytics and consequently, further insightful meaning could be excluded or fail to be captured or misclassified. In other words, although sentiment analysis provides useful insights, due to this identified technical limitation, it falls short of being a silver bullet in social media analysis.
The second challenge refers to the veracity and validity of the original textual dataset in the context of an increase in deceptive and/or fake online information which can somewhat undermine the trustworthiness of sentiment analysis. The malicious intent behind the deceptive and/or fake messages is to manipulate and mislead both businesses and consumers. This means that the points of view expressed in these social media encounters do not necessarily represent authentic sentiments and therefore threaten the accuracy of findings derived from other authentic comments and reviews. Excluding such ânoiseâ or âbiasedâ content from sentiment analysis primarily relies on machine learning algorithms but despite its rigour, it does not completely address the potential for uncertainty in terms of the results.
The third challenge is how hate speech and sarcasm is handled in social media analytics. The ubiquity and anonymity of social media offers an ideal breeding ground to spread hate and sarcasm. Hate speech is characterised by the content and tone of the posting on social media, including provocative words relating to race and/or religion, threats, defamatory statements (e.g. libel or slander) and obscenities. Sarcasm has become a trend in expressing personal emotion via social media postings, and unlike spam filtering, sarcasm is hard to detect in text analysis mainly due to firstly, the ambiguous and rich structure of language which complicates the meaning determination of individual words or terms.
Automated tools for understanding language often miss the deeper meanings and subtle patterns of what people say. Sentiment analysis, which uses algorithms to find opinions on social media, may not always accurately understand the true meaning behind words. This is especially true for the detection of hate speech or sarcasm, which can have harmful effects and require better monitoring systems to quickly identify problematic interactions.
Through the implementation of such a system and the extension of the current English-dominated lexicon into a multi-language classifier and conducting cross-cultural sematic information could improve the accuracy of sentiment analysis outputs and further enhance data-driven decision making in market intelligence.
Associate Professor Dr Nan Jiang is an academic at the School of Management and Marketing at Taylorâs Business School, Faculty of Business and Law, Taylorâs University. Professor Jason James Tuner is the Head Of Southampton Malaysia Business School at the University of Southampton. Taylor’s Business School is the leading private business school in Southeast Asia for Business and Management Studies based on the 2024 QS World University Rankings by Subject and has received the Association to Advance Collegiate Schools of Business (AACSB) accreditation.