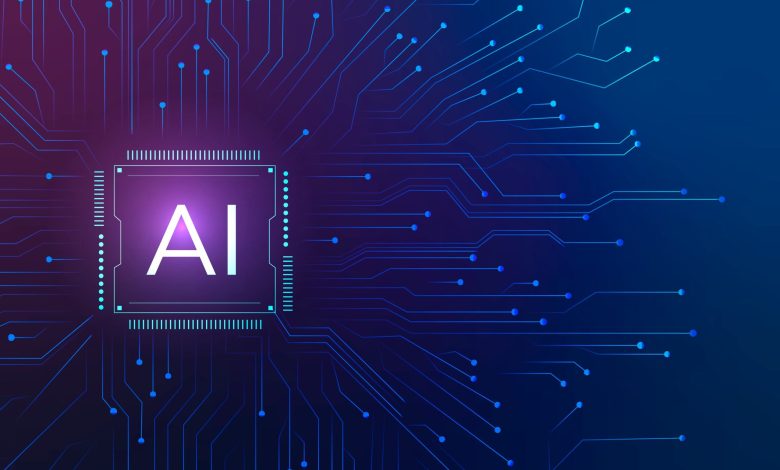
Self-driving cars that zoom seamlessly on the road, smart city and intelligent video surveillance systems, instant machine translation in any language via natural language processing, highly personalised recommendations and increasingly accurate predictions. It seems we’re making tremendous headway in Artificial Intelligence (AI) applications, and it has been all made possible through improvements in Machine Learning (ML).
By infusing AI systems with the ability to continuously learn from the data they’re fed, ML allows these systems to learn and adapt to new data independently, identify patterns, and make decisions and recommendations with minimal human intervention. As such, ML has become a significant competitive differentiator for businesses, including many of today’s leading corporations like Google, Facebook and Uber.
Nevertheless, deploying and scaling ML workloads is not an easy feat since they involve highly mathematical, computational and considerable other complexities. So, it comes as no surprise that only around 35 per cent of organisations have ML models deployed in production. Among the issues facing organisations include:
- Infrastructure: ML production models need to be flexible enough to run anywhere. Whether on-premises or multiple clouds, organisational infrastructure must be able to leverage the security, governance and other critical aspects to boost ML workloads.
- Data Quality: Scarcity of quality and relevant data is perhaps the leading issue organisations face when dealing with Machine Learning models. Another challenge is that data is often spread across multiple clouds and on-prem systems. Noisy, unclean, incomplete and inaccurate data can cause the final ML outcome to make various mistakes, such as incorrect predictions.
- Deployment: There might be inconsistencies in the final ML model due to discrepancies in the initial language, the one with which the model was programmed, and the languages data scientists used while experimenting with the model.
- Governance: Organisations need a better governance framework as they might be unaware of their models, their deployments, and activities, which may result in complexities like redoing, recomputing, model inconsistencies, and other inefficiencies. Governance combined with the monitoring challenges further obscure the visibility of production processes.
- Security: ML models often cannot differentiate between sensitive and insensitive data, which makes end-to-end security of ML servers, data, and production lines an uncompromisable element.
Organisations can choose the services of modern data management companies like Cloudera that not only address the challenges outlined above head-on but also provide additional support, such as full lifecycle integration and scalability, to meet their AI goals. Whether private, public, or multiple cloud environments, Cloudera Machine Learning (CML), Cloudera’s cloud-native machine learning service built for Cloudera Data Platform (CDP), provides a complete portfolio of tools necessary for ML-oriented operations.
CML allows data engineers and scientists to collaborate and perform multi-function analytics and experiments on data located anywhere. This results in better governance and visibility of ML-related workloads and deployments. Business entities can also monitor metrics, such as SLAs, model distribution and uptime, and gear-up Machine Learning projects, from experimentation to production and to finalisation. All of this is packaged into a portable experience that can be easily accessed by multiple team members to provide a consistent experience across an organisation.
Since ML workloads reside in Cloudera Data Cloud, business administrations can ensure smooth workflows and customisation of models already under production, simultaneously keeping control and visibility of all the workloads. In other words, organisations can have hundreds or even thousands of ML models on the production line, and with well-resourcing, they can be auto-scaled in compliance with business needs and model requirements. This further allows the implementation of mission-critical changes while models are under processing.
In short, CML provides an end-to-end machine learning platform for enterprises, leveraging components of CDP in order to deliver a truly seamless experience from data exploration to modelling and launching ML models into production.
Serving thousands of organisations, Cloudera is an industry-leading name known for its exceptional services to customers in building, deploying and managing their ML-related operations and materialising their AI dreams. A name well-established for providing tools, platforms, and expert guidance to its global clients in harnessing the true potential of their businesses using Artificial Intelligence and Machine Learning technologies.
Click on the following link to find out more.