MicroAlgo Develops Quantum Convolutional Neural Network Architecture to Enhance Traditional Computer Vision Performance
MicroAlgo's New Quantum Architecture Has Broad Applications Across Diverse Fields, Including Medicine
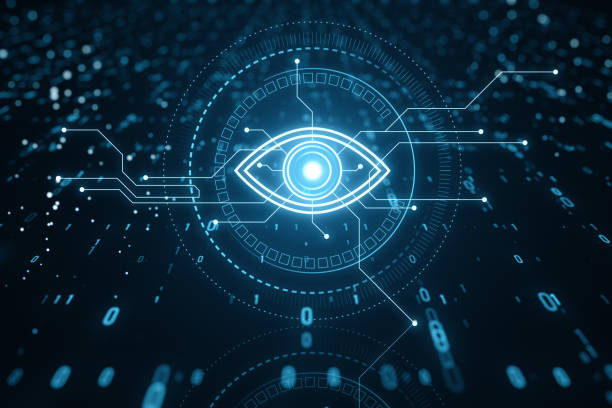
MicroAlgo Inc. have announced their research on quantum visual computing. This investigation involves the integration of quantum computing with classical convolutional neural networks. They are developing a Quantum Convolutional Neural Network (QCNN) architecture to enhance the performance of traditional computer vision tasks using quantum mechanics principles.
The Quantum Convolutional Neural Network (QCNN) architecture is an innovative computational model that cleverly combines the parallelism of quantum computing with the feature extraction capabilities of classical convolutional neural networks. In QCNN, quantum bits (qubits) serve as the basic carrier of information. They use the properties of quantum superposition and entanglement to achieve parallel processing of multiple computational tasks.
At the same time, the QCNN draws inspiration from the structure of classical convolutional neural networks—such as convolution layers, pooling layers, and fully connected layers. As such, QCNN extracts features, reduces dimensions, and classifies image data. These are useful in enhancing both computational speed and image recognition accuracy.
What Computer Vision Can Do as Enhanced by MicroAlgo
Computer vision aims to enable computers to understand and analyse visual data, such as images or videos. This capability is much like the human visual system, involving tasks such as image recognition, object detection, and image segmentation. Quantum computing, with its unique quantum properties like superposition and entanglement, possesses powerful parallel computing capabilities and specialised methods of information processing.
- Data Preparation: Image or video data is collected from multiple channels, then screened and organised to remove low-quality or non-compliant data. The remaining data is preprocessed, including normalising pixel values, resizing images, and correcting and enhancing colours to meet the specifications for subsequent processing.
- Quantum State Encoding: Following specific rules, the preprocessed image features are mapped onto quantum bits and converted into quantum states. By utilising the properties of quantum superposition and entanglement, relationships between features are established, forming a complex network of feature associations.
- Quantum Convolutional Neural Network Processing: The quantum convolutional layer takes advantage of quantum parallelism, using multiple convolutional kernels to extract features represented by quantum states and uncover deeper features. The quantum pooling layer performs dimensionality reduction on the extracted features, retaining key features while alleviating the computational burden in subsequent stages. The quantum fully connected layer analyses the reduced features and classifies them based on quantum state correlations.
- Quantum Measurement and Output: Through appropriate quantum measurement operations, the quantum state results are converted into classical data forms. Outputs such as target categories, locations, and other relevant information are provided, while the entire process is optimised based on application feedback.
Broad Applications in Computer Vision
MicroAlgo’s QCNN architecture has broad application prospects in the field of computer vision. In autonomous driving, QCNN can enable fast and accurate recognition of key elements such as road signs, vehicles, and pedestrians, enhancing the safety and reliability of autonomous driving systems.
In medical imaging analysis, QCNN can achieve rapid and accurate diagnosis of medical images, assisting doctors in disease diagnosis and treatment planning. In security surveillance, QCNN can enable real-time detection and early warning of abnormal behaviour in surveillance videos, improving the efficiency and accuracy of security measures.
Additionally, QCNN can be widely applied in various fields such as smart manufacturing, aerospace, and smart cities, driving technological upgrades and intelligent transformations in related industries.